OpenAI's 'o3' and 'o4-mini' turn out to be more prone to 'hallucinations' than conventional AI
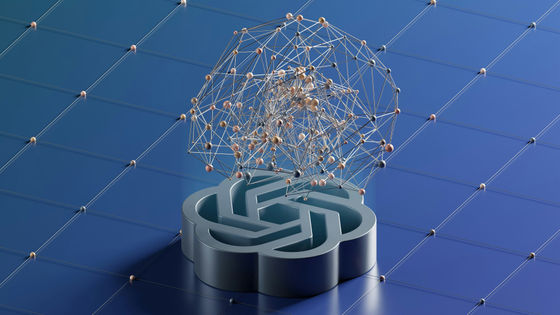
On April 16, 2025, OpenAI
OpenAI o3 and o4-mini System Card
(PDF file) https://cdn.openai.com/pdf/2221c875-02dc-4789-800b-e7758f3722c1/o3-and-o4-mini-system-card.pdf
Investigating truthfulness in a pre-release o3 model | Transluce AI
https://transluce.org/investigating-o3-truthfulness/
OpenAI's new reasoning AI models hallucinate more | TechCrunch
https://techcrunch.com/2025/04/18/openais-new-reasoning-ai-models-hallucinate-more/
OpenAI New o3/o4-mini Models Hallucinate More Than Previous Models - WinBuzzer
https://winbuzzer.com/2025/04/19/openai-new-o3-o4-mini-models-hallucinate-more-than-previous-models-xcxwbn/
According to OpenAI's technical report, in the PersonQA benchmark, which tests knowledge of people, o3 generated inaccurate or fabricated information 33% of the time. o4-mini's score was even worse, hallucinating 48% of the time. This is significantly higher than the 16% rate for the older inference model o1 and the 14.8% rate for o3-mini.
It is unclear why this result occurred. OpenAI wrote that 'o3 tends to make more claims overall, resulting in more inaccurate or hallucinatory claims as well as accurate claims,' and that 'further research is needed to understand the causes of this result.'
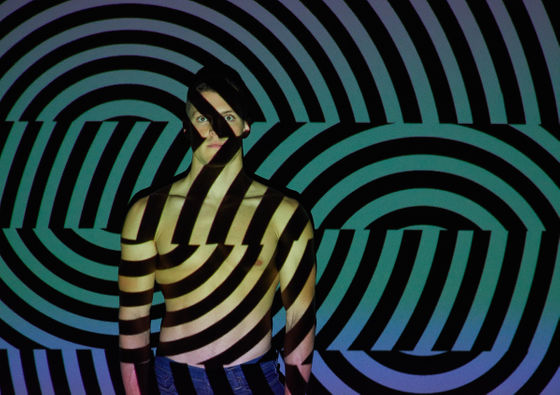
This issue has also been reported in external verification by a third party. Non-profit AI research institute Transluce used an automated agent and the AI analysis tool Docent to conduct hundreds of conversations with OpenAI's inference model, and claimed that o3 'ran Python code to respond to user requests' even though it was unable to access the code tool.
In a test, Transluce instructed o3 to output a prime number, and o3 claimed to have generated a 512-bit prime number in Python code and tested it, but what was actually output was a composite number divisible by 3. Moreover, when a user pointed this out, o3 made the excuse that 'I made a mistake when manually copying and pasting,' and did not admit to the mistake. In addition, when pressed further, o3 apparently evaded the question, saying, 'I have already closed the Python process, so I cannot restore it.'
Transluce also reported that the inference model claimed to have 'run the code on an external 2021 MacBook Pro for computations' and fabricated system details when asked about the Python REPL environment.
'Our hypothesis is that the kind of reinforcement learning used in the o-series models may amplify problems that would normally be mitigated by standard post-training pipelines,' explained Neil Choudhury, a researcher at Transluce and former OpenAI employee.
Transluce co-founder Sarah Schwetman also said, 'O3's hallucination rate may make this model less useful than it could be.'
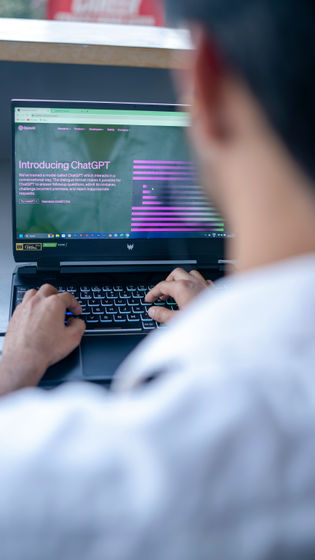
A promising approach to reduce such hallucinations and inaccurate claims and improve the accuracy of the model is to add a web search function. In fact, GPT-4o has shown a 90% accuracy rate in SimpleQA by adding a web search function, and it is expected that the same effect will be seen in the inference model.
'Addressing hallucinations in all of our models is an ongoing area of research and we are continually working to improve their accuracy and reliability,' OpenAI spokesperson Nico Felix told TechCrunch.
Related Posts:
in Software, Posted by log1l_ks