AI misses illnesses in black people and women, analysis of chest x-rays reveals
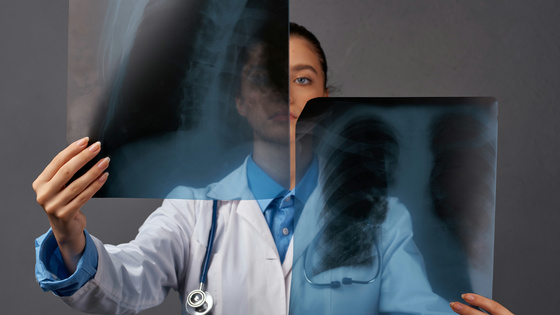
A research team at the University of California, Los Angeles examined the accuracy of disease detection using an AI model called ' CheXzero ,' developed at Stanford University in 2022, and reported that there was a bias in disease detection in black and female patients.
Demographic bias of expert-level vision-language foundation models in medical imaging | Science Advances
https://www.science.org/doi/10.1126/sciadv.adq0305
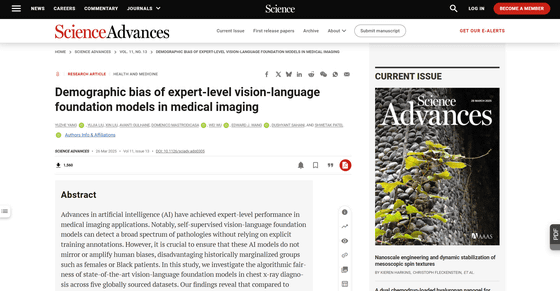
AI models miss disease in Black and female patients | Science | AAAS
https://www.science.org/content/article/ai-models-miss-disease-black-female-patients
CheXzero is a model used to detect disease in chest X-rays. When the research team tested it on a subset of 666 chest X-rays, they found that black patients, female patients, and patients under the age of 40 were more likely to fail to detect diseases diagnosed by doctors.
The researchers found that in half of cases, conditions such as cardiac hypertrophy were not detected when patients were black women. These issues were also observed in datasets from other regions, including Spain and Vietnam.
To explore the source of bias, the research team tested CheXzero to see if it could predict a patient's gender, age, and race from chest X-ray images. As a result, the AI was able to identify race with about 80% accuracy, while a similar test by an experienced radiologist had a success rate of only about 50% at best. This led the researchers to say that 'there may be hidden signals in radiological images that humans cannot visually identify.'
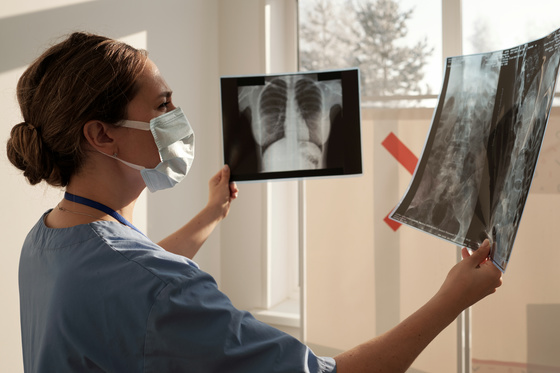
To mitigate bias, the team purposefully provided the patient's race, sex, and age information along with the X-ray images to the AI, which cut the rate of 'missed' diagnoses in half, but only for some diseases.
The research team noted that the dataset used to train CheXzero was overrepresented in men, the 40-80 age group, and white patients, and called for a more diverse dataset.
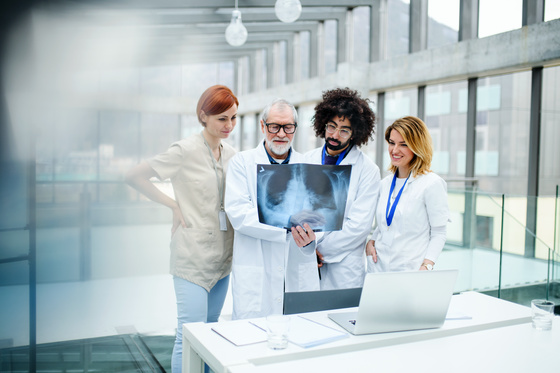
'What's clear is that it's very difficult to mitigate bias in AI,' said Judy Gikoya, a radiologist at Emory University. She advocates testing AI to identify flaws and correct them on small, diverse data sets first. 'Humans need to be involved in diagnosis. AI can't be left to its own devices,' she said.
Related Posts: